Back to all blogs
Navigating AI Risk Management: A Simple Guide
Navigating AI Risk Management: A Simple Guide
Sep 1, 2024
|
8 min read
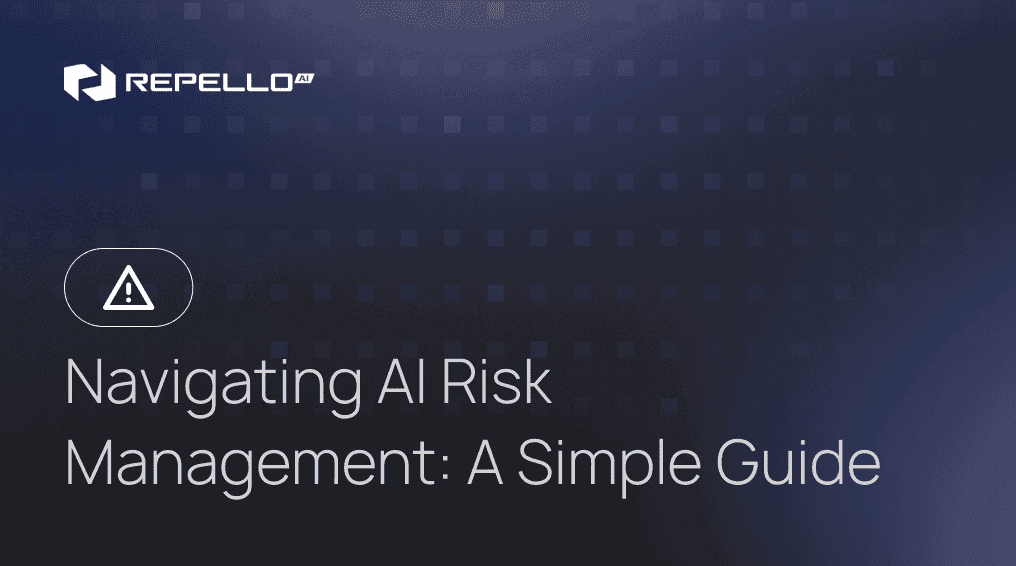
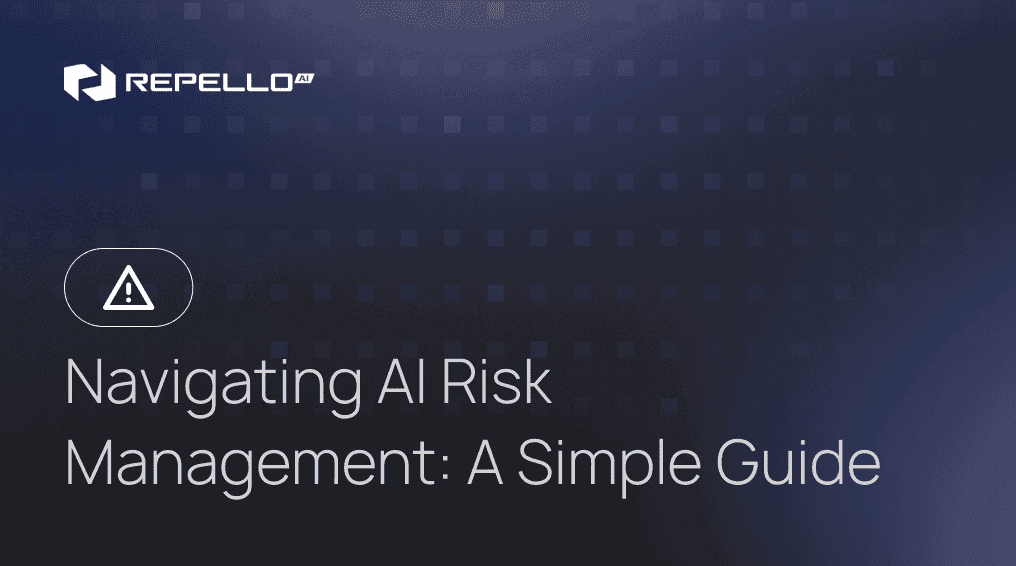
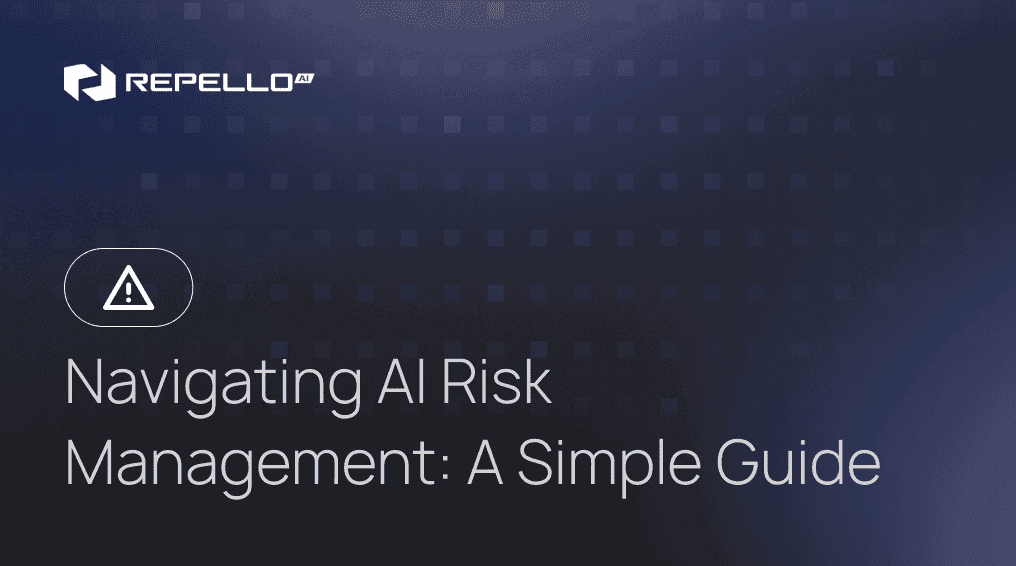
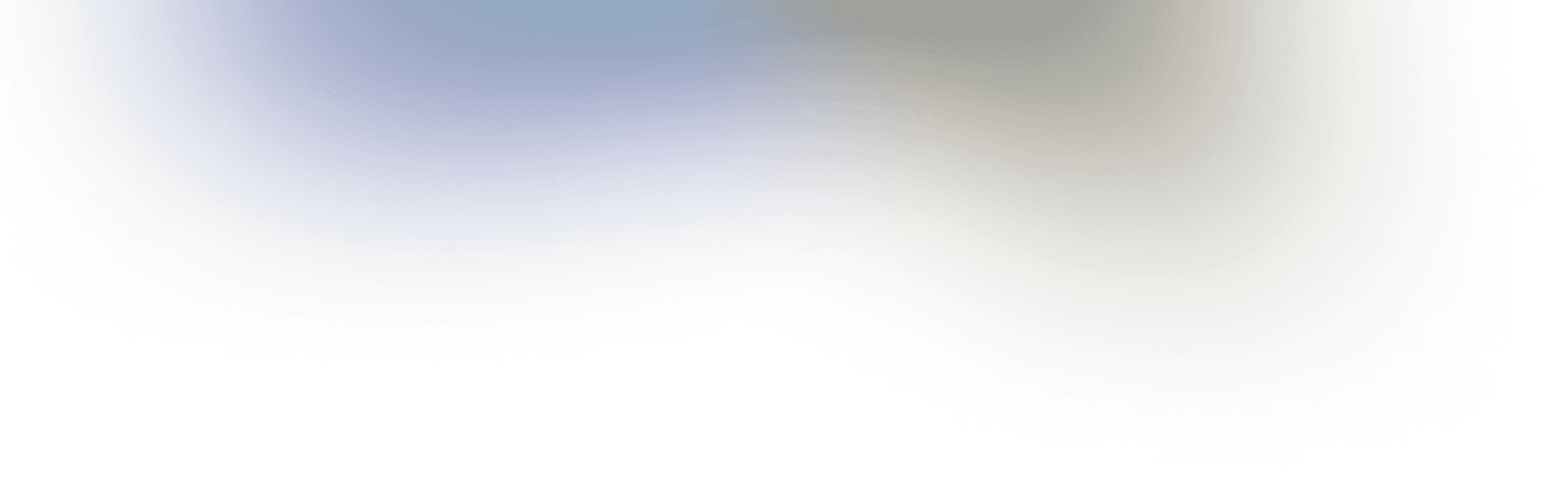
What is AI Risk Management?
As a tech leader, you're likely grappling with the rapid rise of artificial intelligence in your organization. While AI promises groundbreaking innovations, it also introduces new risks that demand your attention.
From data privacy concerns to algorithmic bias, navigating the complex landscape of AI risk management can feel overwhelming.
AI risk management involves identifying, assessing, and mitigating potential risks associated with AI systems to ensure their safe and responsible deployment.
AI Governance vs AI Risk Management
AI governance is a broad field that sets the frameworks, rules, and standards guiding AI development, ensuring safety, fairness, and respect for human rights. Within this discipline, AI risk management is a targeted process that focuses on identifying and mitigating specific vulnerabilities and threats to safeguard AI systems from potential harm.
How does AI risk differ from traditional software risk?
AI risk differs from traditional software risk because AI models rely heavily on data rather than just code, learning logic from data instead of predefined instructions. This makes testing more complex, as users cannot directly observe the model's logic. Rigorous testing across diverse datasets is necessary, beyond simple input-output checks.
Additionally, AI models are evaluated with different metrics, like accuracy, and face unique challenges, such as bias and fairness, that require specialized testing approaches to mitigate risks in various AI tasks and modalities.
Is There a Need for AI Risk Management?
A recent study by McKinsey revealed that 96% of leaders believe that adopting generative AI increases the likelihood of a security breach. Despite this concern, the study also found that only 24% of current generative AI projects have proper security measures.
AI risk management can bridge this gap, enabling organizations to fully leverage AI systems while ensuring that data, ethics, and security are not compromised.
Understanding AI Risk Management
AI systems are exposed to several significant risks that organizations must address:
Data Risks: These encompass issues related to data security, such as unauthorized access or loss, data privacy involving the handling of sensitive personal information, and data integrity, which concerns the reliability of the training data used.
Model Risks: Risks in this category include adversarial attacks, where inputs are manipulated to deceive the model, prompt injections that target large language models to spread misinformation, challenges with model interpretability which make understanding model decisions difficult, and supply chain attacks that exploit vulnerabilities in third-party components.
Operational Risks: Key operational risks involve model drift, where performance degrades over time, sustainability issues related to maintaining and updating AI systems, integration challenges with existing infrastructure, and a lack of accountability due to insufficient oversight.
Ethical and Legal Risks: These include a lack of transparency, which can erode public trust, noncompliance with regulatory requirements leading to legal penalties, algorithmic biases that result in discriminatory outcomes, ethical dilemmas related to privacy and human rights, and a lack of explainability, which makes it difficult to justify AI decisions.
If not properly managed, these risks can lead to severe consequences such as financial losses from a denial of wallet attack and reputational damage, along with regulatory penalties from RAG poisoning.
Key Elements of Effective AI Risk Management
Risk Identification: Systematically recognize potential AI-related threats, including data privacy concerns, bias issues, and compliance risks.
Risk Assessment: Evaluate the likelihood and potential impact of identified risks on individuals, organizations, and ecosystems.
Risk Mitigation: Develop and implement strategies to address and minimize identified risks, such as improving data quality and enhancing AI model transparency.
Continuous Monitoring: Regularly assess AI system performance and emerging risks through AI Red Teaming to ensure ongoing safety and effectiveness.
Standardizing AI Risk Management Practices
Much like other established forms of risk management, AI risk management is quickly becoming a standard practice across industries.
By implementing such frameworks, organizations can proactively mitigate risks and maximize the benefits of AI technologies.
Exploring key differences between popular AI Risk Management Frameworks:
1. NIST AI Risk Management Framework (AI RMF)
Purpose: To provide a comprehensive framework for managing risks associated with AI systems, focusing on trustworthiness and ethical considerations.
Scope: Applicable across various sectors, emphasizing governance, accountability, and transparency throughout the AI lifecycle.
Implementation Guidance: Provides a flexible approach that can be tailored to an organization’s specific needs and risk tolerance. Encourages voluntary adoption and integration into existing risk management processes.
Target Audience: Organizations across various sectors, including public and private entities involved in AI development and deployment.
2. Google’s Secure AI Framework (SAIF)
Purpose: To enhance the security of AI systems by addressing specific vulnerabilities and threats.
Scope: Focuses on integrating security measures into the AI development process, addressing issues like model theft and data poisoning.
Implementation Guidance: Focuses on practical implementation of security measures and continuous adaptation to emerging threats. Promotes industry collaboration to enhance security standards.
Target Audience: Primarily aimed at organizations developing AI technologies and seeking to enhance their security posture.
3. EU AI Act
Purpose: To regulate AI within the EU, ensuring safe and trustworthy AI applications while protecting fundamental rights.
Scope: Introduces a risk-based categorization system for AI applications, establishing harmonized rules across member states.
Implementation Guidance: Establishes clear compliance requirements for organizations operating within the EU, influencing global standards. Mandates adherence to regulations, with penalties for non-compliance.
Target Audience: Companies and organizations operating within the EU, particularly those developing or deploying AI systems.
4. OWASP Top 10 for AI
Purpose: To identify and prioritize the most critical security risks associated with AI systems.
Scope: Provides a list of the top ten risks, offering guidance on how to mitigate these vulnerabilities effectively.
Implementation Guidance: Provides actionable recommendations for developers and organizations to mitigate identified risks. Developed through community input, ensuring relevance to current security challenges.
Target Audience: developers, security professionals, and organizations involved in AI system development and deployment.
Read more on our OWASP Top 10 for LLMs explained guide for CISOs.
Conclusion
Each framework serves a distinct purpose in AI risk management:
NIST AI RMF focuses on comprehensive risk management and ethical considerations.
Google SAIF emphasizes AI security and proactive measures.
The EU AI Act provides regulatory compliance and harmonization within the EU.
OWASP Top 10 for AI identifies critical risks and offers mitigation strategies.
As a CISO, CTO, or tech leader, your role is pivotal in guiding your organization through the ever-evolving landscape of AI risks. It's essential to continually reassess your strategies, conduct Red Team exercises on your AI applications, and remain agile to adapt to emerging challenges.
What is AI Risk Management?
As a tech leader, you're likely grappling with the rapid rise of artificial intelligence in your organization. While AI promises groundbreaking innovations, it also introduces new risks that demand your attention.
From data privacy concerns to algorithmic bias, navigating the complex landscape of AI risk management can feel overwhelming.
AI risk management involves identifying, assessing, and mitigating potential risks associated with AI systems to ensure their safe and responsible deployment.
AI Governance vs AI Risk Management
AI governance is a broad field that sets the frameworks, rules, and standards guiding AI development, ensuring safety, fairness, and respect for human rights. Within this discipline, AI risk management is a targeted process that focuses on identifying and mitigating specific vulnerabilities and threats to safeguard AI systems from potential harm.
How does AI risk differ from traditional software risk?
AI risk differs from traditional software risk because AI models rely heavily on data rather than just code, learning logic from data instead of predefined instructions. This makes testing more complex, as users cannot directly observe the model's logic. Rigorous testing across diverse datasets is necessary, beyond simple input-output checks.
Additionally, AI models are evaluated with different metrics, like accuracy, and face unique challenges, such as bias and fairness, that require specialized testing approaches to mitigate risks in various AI tasks and modalities.
Is There a Need for AI Risk Management?
A recent study by McKinsey revealed that 96% of leaders believe that adopting generative AI increases the likelihood of a security breach. Despite this concern, the study also found that only 24% of current generative AI projects have proper security measures.
AI risk management can bridge this gap, enabling organizations to fully leverage AI systems while ensuring that data, ethics, and security are not compromised.
Understanding AI Risk Management
AI systems are exposed to several significant risks that organizations must address:
Data Risks: These encompass issues related to data security, such as unauthorized access or loss, data privacy involving the handling of sensitive personal information, and data integrity, which concerns the reliability of the training data used.
Model Risks: Risks in this category include adversarial attacks, where inputs are manipulated to deceive the model, prompt injections that target large language models to spread misinformation, challenges with model interpretability which make understanding model decisions difficult, and supply chain attacks that exploit vulnerabilities in third-party components.
Operational Risks: Key operational risks involve model drift, where performance degrades over time, sustainability issues related to maintaining and updating AI systems, integration challenges with existing infrastructure, and a lack of accountability due to insufficient oversight.
Ethical and Legal Risks: These include a lack of transparency, which can erode public trust, noncompliance with regulatory requirements leading to legal penalties, algorithmic biases that result in discriminatory outcomes, ethical dilemmas related to privacy and human rights, and a lack of explainability, which makes it difficult to justify AI decisions.
If not properly managed, these risks can lead to severe consequences such as financial losses from a denial of wallet attack and reputational damage, along with regulatory penalties from RAG poisoning.
Key Elements of Effective AI Risk Management
Risk Identification: Systematically recognize potential AI-related threats, including data privacy concerns, bias issues, and compliance risks.
Risk Assessment: Evaluate the likelihood and potential impact of identified risks on individuals, organizations, and ecosystems.
Risk Mitigation: Develop and implement strategies to address and minimize identified risks, such as improving data quality and enhancing AI model transparency.
Continuous Monitoring: Regularly assess AI system performance and emerging risks through AI Red Teaming to ensure ongoing safety and effectiveness.
Standardizing AI Risk Management Practices
Much like other established forms of risk management, AI risk management is quickly becoming a standard practice across industries.
By implementing such frameworks, organizations can proactively mitigate risks and maximize the benefits of AI technologies.
Exploring key differences between popular AI Risk Management Frameworks:
1. NIST AI Risk Management Framework (AI RMF)
Purpose: To provide a comprehensive framework for managing risks associated with AI systems, focusing on trustworthiness and ethical considerations.
Scope: Applicable across various sectors, emphasizing governance, accountability, and transparency throughout the AI lifecycle.
Implementation Guidance: Provides a flexible approach that can be tailored to an organization’s specific needs and risk tolerance. Encourages voluntary adoption and integration into existing risk management processes.
Target Audience: Organizations across various sectors, including public and private entities involved in AI development and deployment.
2. Google’s Secure AI Framework (SAIF)
Purpose: To enhance the security of AI systems by addressing specific vulnerabilities and threats.
Scope: Focuses on integrating security measures into the AI development process, addressing issues like model theft and data poisoning.
Implementation Guidance: Focuses on practical implementation of security measures and continuous adaptation to emerging threats. Promotes industry collaboration to enhance security standards.
Target Audience: Primarily aimed at organizations developing AI technologies and seeking to enhance their security posture.
3. EU AI Act
Purpose: To regulate AI within the EU, ensuring safe and trustworthy AI applications while protecting fundamental rights.
Scope: Introduces a risk-based categorization system for AI applications, establishing harmonized rules across member states.
Implementation Guidance: Establishes clear compliance requirements for organizations operating within the EU, influencing global standards. Mandates adherence to regulations, with penalties for non-compliance.
Target Audience: Companies and organizations operating within the EU, particularly those developing or deploying AI systems.
4. OWASP Top 10 for AI
Purpose: To identify and prioritize the most critical security risks associated with AI systems.
Scope: Provides a list of the top ten risks, offering guidance on how to mitigate these vulnerabilities effectively.
Implementation Guidance: Provides actionable recommendations for developers and organizations to mitigate identified risks. Developed through community input, ensuring relevance to current security challenges.
Target Audience: developers, security professionals, and organizations involved in AI system development and deployment.
Read more on our OWASP Top 10 for LLMs explained guide for CISOs.
Conclusion
Each framework serves a distinct purpose in AI risk management:
NIST AI RMF focuses on comprehensive risk management and ethical considerations.
Google SAIF emphasizes AI security and proactive measures.
The EU AI Act provides regulatory compliance and harmonization within the EU.
OWASP Top 10 for AI identifies critical risks and offers mitigation strategies.
As a CISO, CTO, or tech leader, your role is pivotal in guiding your organization through the ever-evolving landscape of AI risks. It's essential to continually reassess your strategies, conduct Red Team exercises on your AI applications, and remain agile to adapt to emerging challenges.
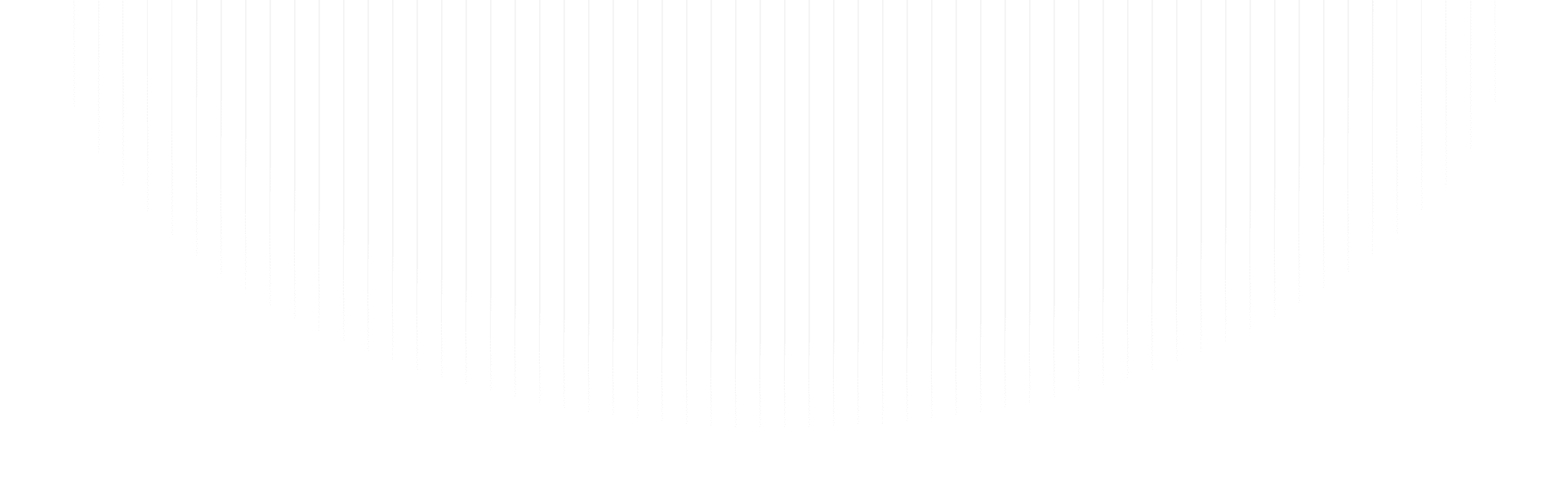
You might also like
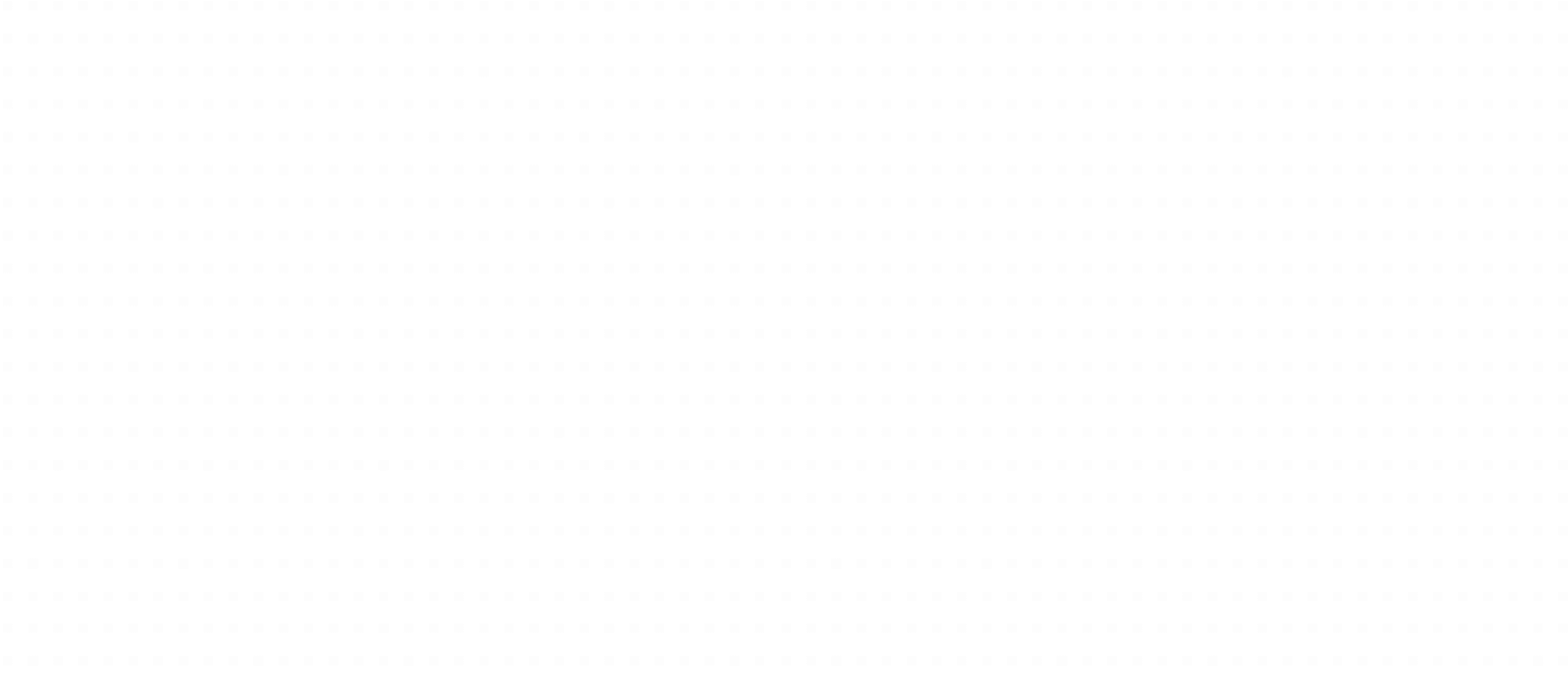
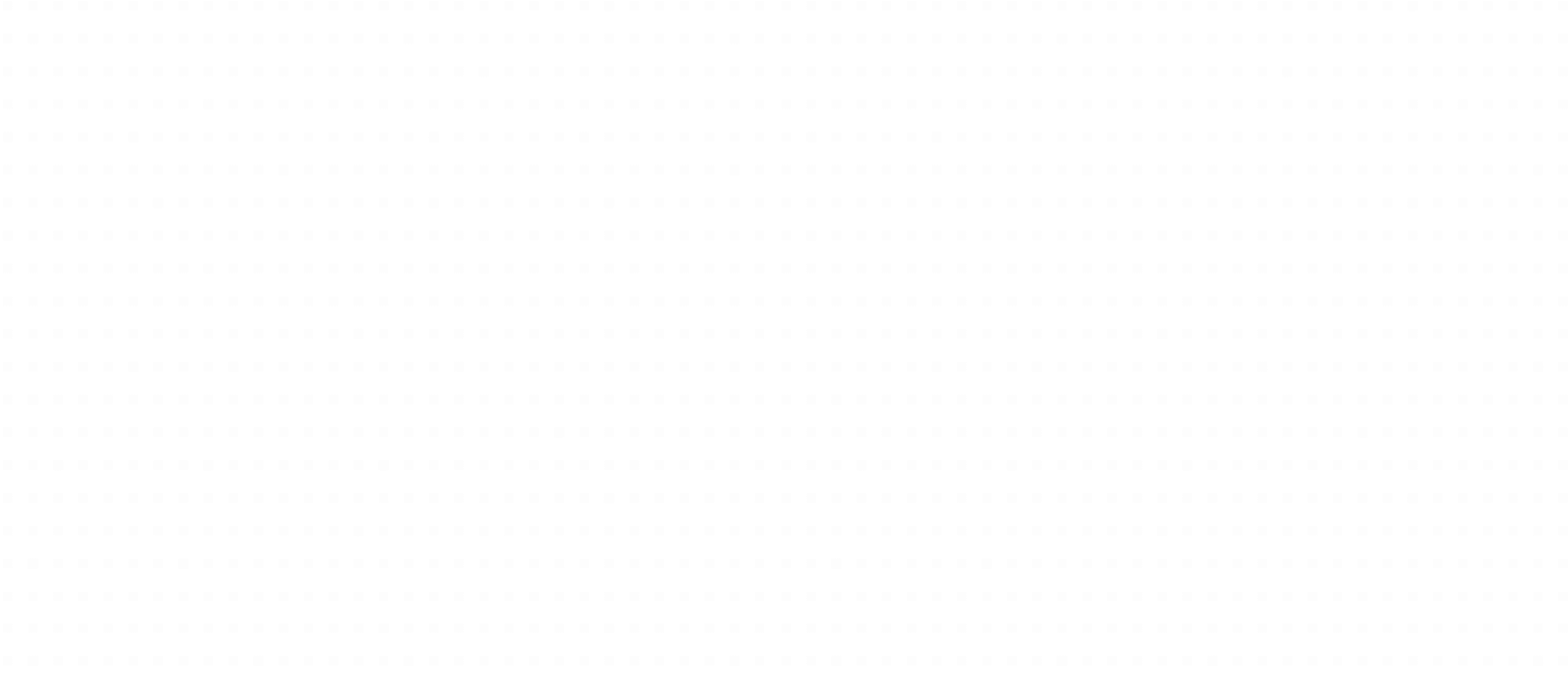
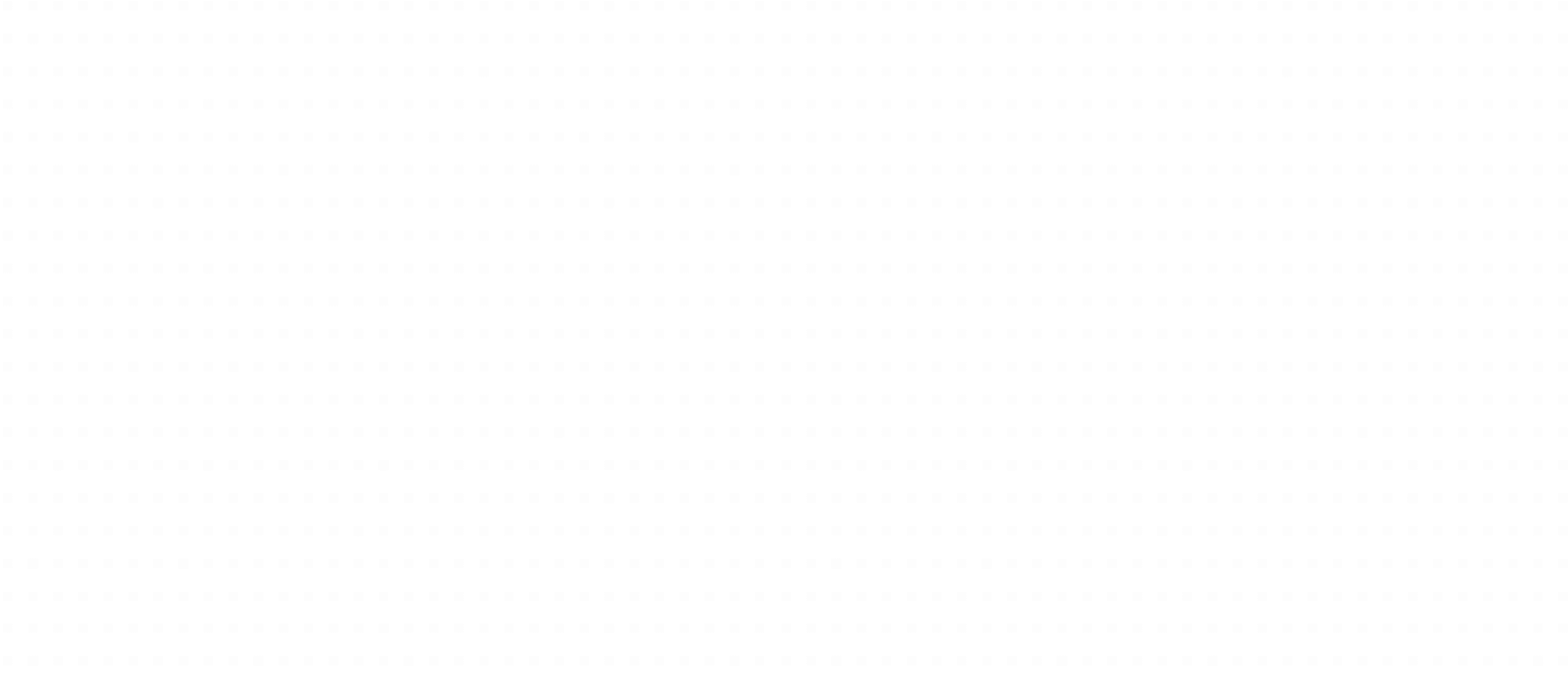